Modern multivariate and time series analyses go beyond the classical normality assumption by modelling data that could combine binary, categorical, extreme and heavy-tailed distributions. Dependence is modeled non-linearly, often in terms of copula functions or stochastic representations. Models for multivariate extremes arise from asymptotic limits. Characterization and modelling of dependence among extremes as well as estimation of probabilities of rare events are topics of on-going research. Advances in high-dimensional multivariate modelling have been achieved by the use of vine pair-copula constructions. Areas of application include biostatistics, psychometrics, genetics, machine learning, econometrics, quantitative risk management in finance and insurance, hydrology and geoscience.
Recent Highlights
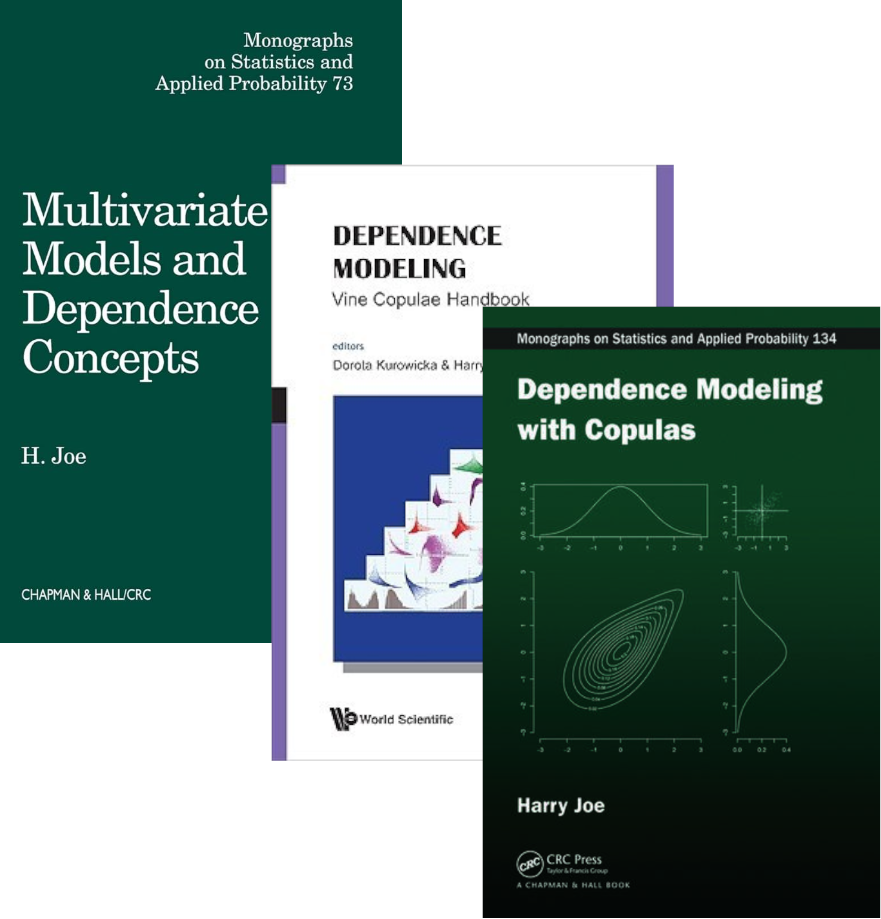
Multivariate Modeling with Copulas
H. Joe
This foundational sequence of books by Professor Joe provides a deep dive into the advances that have taken place in the field of dependence modeling during the last 30 years, including various multivariate non-normal distributions for modeling binary, count, ordinal, and extreme-value response data. Copula modeling of high-dimensional data, including Vine Copulas, are a special focus of the series. It also discusses other multivariate constructions and parametric copula families that have different tail properties and presents extensive material on dependence and tail properties to assist in copula model selection.