The Bayesian paradigm for statistical inference uses expert knowledge, formulated in terms of probability distributions of unknown parameters of interest. These distributions, called prior distributions, are combined with data to provide new information about parameters, via new parameter distributions called posterior distributions. One research theme centers on devising new Bayesian methodologies, i.e., new statistical models with which Bayesian inferences can provide particular scientific insight. Quantifying the statistical properties of such methods and contrasting with non-Bayesian alternatives is an active area of research. Bayesian methods can lead to computational challenges, and another research theme centers on efficient computation of Bayesian solutions. The development of computational techniques for determining posterior distributions, such as Monte Carlo methods, is a rich area of research activity, with particular emphasis on Markov Chain Monte Carlo methods and sequential Monte Carlo methods.
Recent Highlights
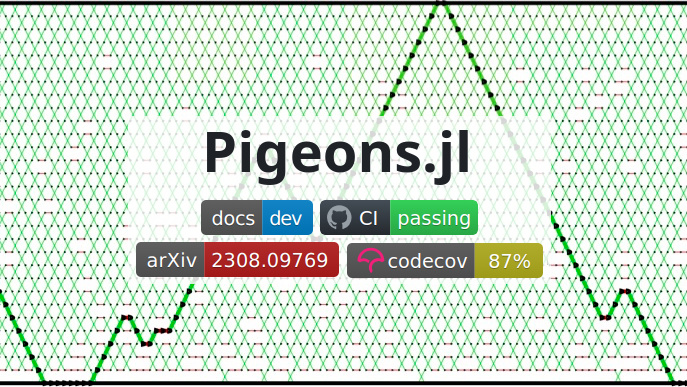
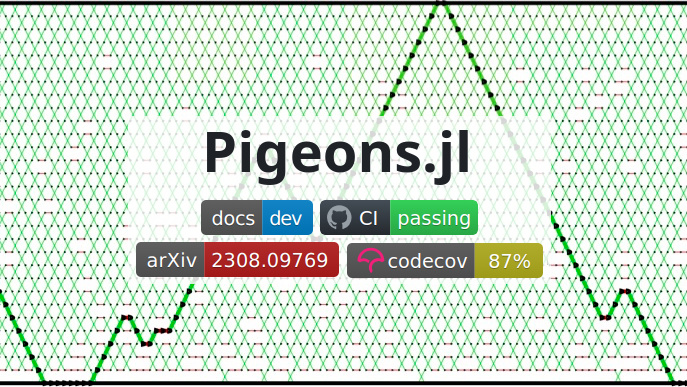
Pigeons.jl: Distributed Parallel Tempering
A. Bouchard-Côté, T. Campbell
Pigeons is a Julia package used to estimate expectations of challenging posterior distributions, and more broadly, approximately solve Lebesgue integration problems. Pigeons can be used in a multi-threaded context, and/or distributed over hundreds or thousands of MPI-communicating machines. Principal Investigators Bouchard-Côté and Campbell (in collaboration with P. Gagnon at U de M and L. Wang at SFU) were awarded a 2025 CANSSI Collaborative Research Teams grant for this project.
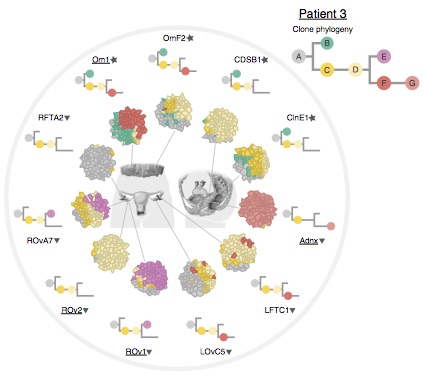
Probabilistic modelling of the evolutionary dynamics and phylogeny of cancer
A. Bouchard-Côté, A. Roth
Proliferating cancer cells, in which DNA repair mechanisms are disrupted, accumulate mutations at a much faster rate than healthy cells do. This leads to the emergence of an evolutionary process inside the tumour. A current research frontier is the characterization of the evolutionary dynamics and phylogenies within individual cancer tumours, where multiple sub-populations of cancer cells acquire differentiating sets of mutations.
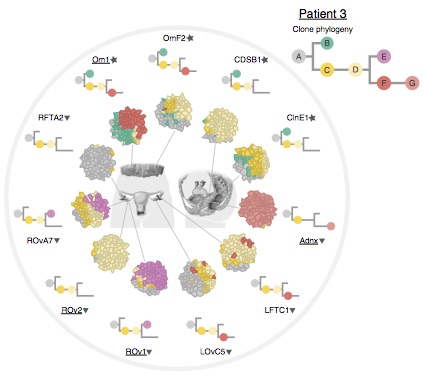